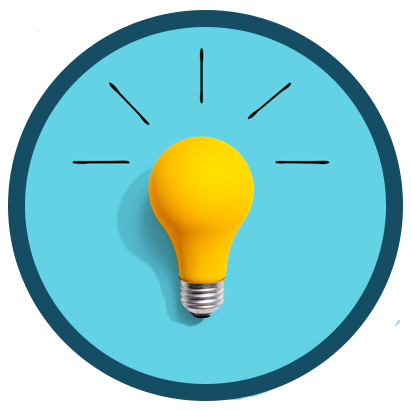
While many generative AI use cases emphasize increased productivity and labor savings, with the right approach, a smart business can improve the quality of its workforce, rather than downsizing it, building a foundation for an innovative, growth-oriented future.
Gen AI Can Help Your Staff Work Smarter, not Just Faster
There’s no doubt that generative AI is changing the way everyone works. From increasing productivity at all skill levels to automating repetitive and mundane tasks, AI will impact nearly every employment function. While such monumental shifts can lead to widespread anxiety about job losses and rapid changes in expectations, gen AI presents opportunities to help your staff increase their skills across a variety of capacities, particularly through the use of LLMs in training and everyday job functions.
Take coding, for example. Foundation models can generate code from plain-language text prompts, and can interpret, validate, translate, and revise code with further prompting in a conversational cycle. As a result, coding is democratized, allowing less-experienced, less-trained developers to produce more advanced code than would otherwise be possible, with increased productivity. So far, so good. What’s even better is that an inexperienced developer has the chance to learn from the process, building new knowledge and skills in real time, with every LLM interaction. Suddenly, that developer is not quite so inexperienced.
Operations support provides another great example. In an integration like Cascadeo AI’s composite AI approach, which merges AI with human expertise, support engineers receive AI-derived analysis, validation, and remediation recommendations with each system alert, allowing them to eliminate the triage work that accounts for up to 80% of event response time, dramatically increasing ticket response efficiency and allowing a Tier 2 engineer to perform like Tier 3. The AI integration’s augmented alerts provide them with the knowledge to respond at a higher level to every future ticket, as well, helping build knowledge about causes and remediations even for novel events.
Generative AI’s relationship with data is also changing the nature of research in a variety of ways. The ingestion and processing of massive quantities of data are, of course, defining features of foundation models. For researchers, this means that information that may have once taken a lifetime to curate and comprehend can be summarized in plain language by an LLM in a single action. LLMs implementations are providing researchers with analysis, visualization, and pattern recognition among large data collections, as well, helping to accelerate the learning process that makes up the core of research in most disciplines. Because foundation models can be fine-tuned and specialized, researchers can direct LLMs to analyze precisely-defined data sets in myriad ways as their ideas develop and evolve. This serves humanity, for example in a case where researchers at UC San Diego used generative AI to design antibiotics that are effective against drug-resistant bacteria; it also serves the researchers themselves, freeing them up to explore new ideas and knowledge and to test theoretical concepts with synthetic data that would have required years of model development and data collection with traditional methods.
LLMs can also be deployed as powerful learning tools via their interpretation and translation functions, democratizing complicated ideas and jargon by reframing and summarizing large bodies of technical information in plain language. Through this process, employees with limited access to education and training can overcome the barriers presented by academic and professionalization standards to obtain knowledge typically reserved for those who’ve had the privilege of many years of study. While most publicly available LLMs do require considerable fact-checking, the fact-checking process, as well, serves an educational purpose for an employee who is eager to grow their knowledge.
Another AI-created learning opportunity occurs simply when employees focus more thoroughly on the higher-skill, more creative aspects of their jobs or are enabled to devote time to professional development due to work hours saved using AI to complete rote tasks. A junior-level attorney can leave the drafting of standard contracts to an LLM and instead focus on solving client problems and brushing up on case law, for example. A lab director can engage an LLM to analyze data, draft reports, and manage databases, freeing up time to think more intensively about R&D. A professor can offload syllabus drafting and instead focus more deeply on understanding what modern students need to learn effectively.
Embracing this forward-thinking use of AI demands leadership devoted to talent development and retention. While many generative AI use cases emphasize increased productivity and labor savings, with the right approach, a smart business can improve the quality of its workforce, rather than downsizing it, building a foundation for an innovative, growth-oriented future.